Evaluating AI Agents: Metrics and Best Practices
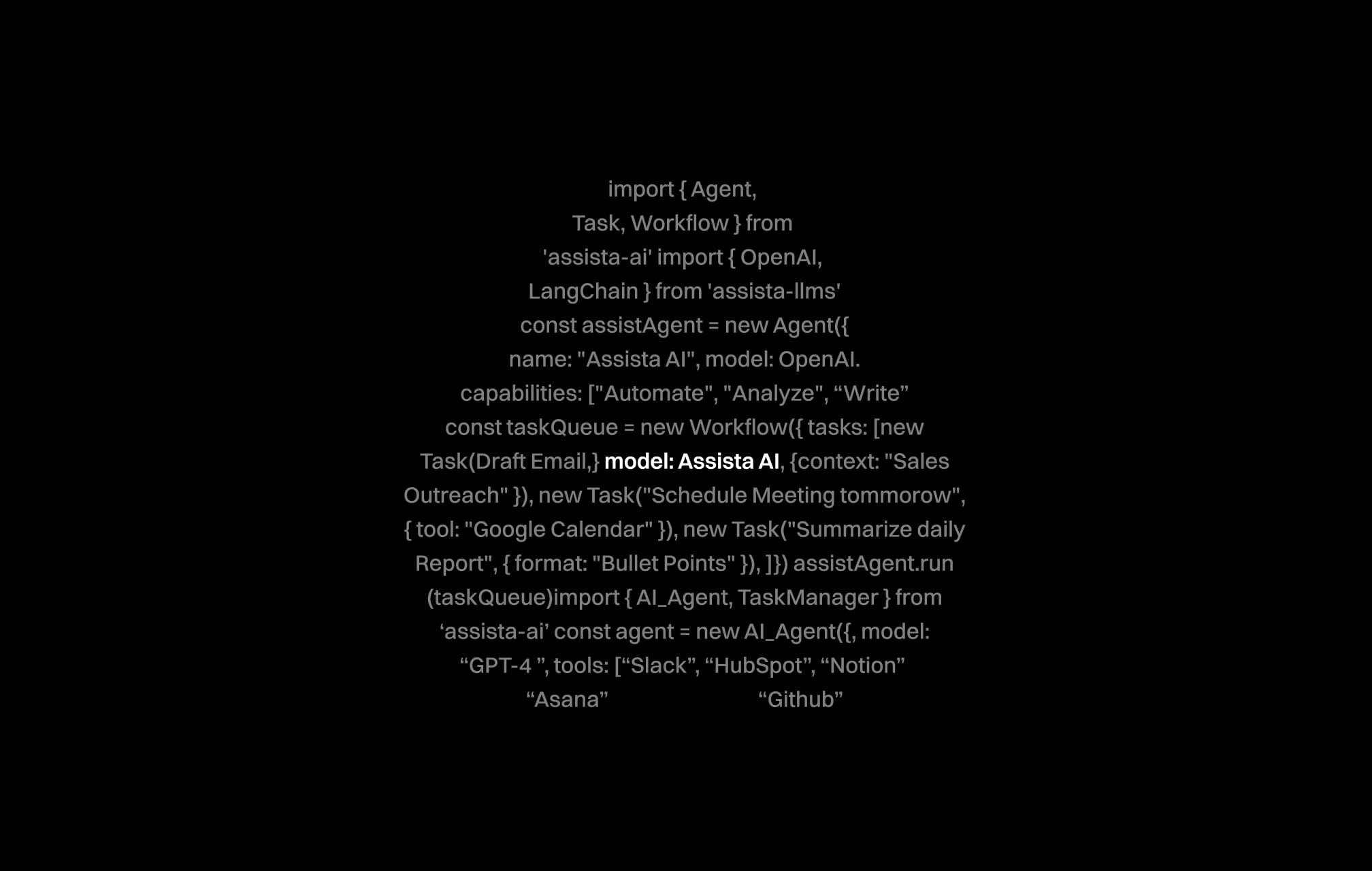
Understanding the Importance of AI Agent Evaluation
When you work with AI agents, knowing their performance details is vital. For anyone considering effective ai agent evaluation, understanding its impact can transform how you manage workflows and deliver results. You might be questioning how to measure an AI agent's success, and why these metrics matter. This article explains how comprehensive evaluation not only improves reliability and performance but also makes it easier to harness AI power for your operations. We will explore crucial metrics, evaluation best practices, data-driven strategies, and even real-world examples that demonstrate how robust assessments can drive better business outcomes.
In today’s digital age, measuring performance accurately provides insights that can reduce errors, boost productivity, and enhance user experiences. As you read on, you will discover methods to quantify success, planned frameworks, and case studies that illustrate these concepts in practice. Transitioning from theory to real applications, you’ll learn how to incorporate these principles into your existing setups.
Key Metrics for Measuring AI Agent Performance
Understanding which metrics to track is the first step toward a successful evaluation strategy. You need to focus on metrics that shed light on speed, accuracy, efficiency, and user outcomes. Robust ai agent evaluation involves looking at quantitative data and qualitative feedback to get a complete picture of performance.
Defining Relevant Metrics
Metrics play a critical role in assessing how well your AI agents perform. For example, measuring response time, accuracy in task execution, and error rates can be a strong indicator of how the system is functioning. In addition, monitoring uptime and latency is essential when numerous actions run across platforms like Gmail, Trello, or Google Sheets. Data-driven metrics help you adjust strategies quickly and ensure your agents meet established benchmarks.
Performance, Accuracy, and Reliability
To assess performance, tracking response speed and error frequency provides clear indicators of where improvements are necessary. Consider the essential performance metrics:
- Response Time: How quickly the AI agent executes the task.
- Error Rate: The frequency of mistakes made during task execution.
- Task Completion Rate: The percentage of tasks finished without human intervention.
Moreover, user satisfaction scores supplement these technical measures. By combining them, you get a holistic view of your AI agent's performance. For further insights into performance standards, the National Institute of Standards and Technology offers valuable research on technology evaluation.
Best Practices in AI Agent Evaluation
Establishing a systematic evaluation framework is essential. Best practices in ai agent evaluation ensure your systems not only function properly but also continue to improve over time. A structured approach provides actionable insights that help you fix issues before they disrupt daily operations.
Establishing a Clear Evaluation Framework
The first step in the process is creating a step-by-step method to evaluate your AI agents regularly. Clarity in objectives and processes leads to more consistent results. Key elements of the framework include:
- Defining clear performance benchmarks and expectations.
- Outlining procedures for regular performance checks and audits.
- Implementing robust reporting systems that capture every metric.
- Incorporating both automated tests and manual reviews, especially for complex workflows.
This approach not only identifies weak points but also ensures that continuous improvements are effectively implemented.
Data-Driven Strategies for Continuous Improvement
You should always leverage data when determining the effectiveness of your AI agents. Using analytics tools and dashboards simplifies the process of tracking performance over time. Incorporating data analysis enables you to:
- Monitor real-time performance and adapt quickly.
- Establish benchmarks based on historical data.
- Identify emerging patterns and potential issues early.
- Align the evaluation metrics with overall business objectives.
Integrating feedback from user experiences and technical logs creates a comprehensive perspective on operational effectiveness. In addition, revisiting these benchmarks periodically can help you synchronize the evaluation system with evolving technology trends. For more detailed strategies, check out related content on the integrations page and visit the blog.
Real-world Examples and Case Studies
Applying measurement techniques in real-world scenarios confirms the value of robust ai agent evaluation. Many organizations have embraced performance metrics to optimize automated workflows and drive actionable business insights. Learning from case studies can offer you clarity on both common challenges and effective solutions.
Case Study: Multi-tool Automation with Assista
Consider a digital agency using Assista to automate email communication, task management, and social media posting. The agency leveraged metrics such as response time and task completion rate to evaluate the AI agents working with Gmail, Trello, and LinkedIn. They observed a 30% boost in efficiency after refining their evaluation methods. Through this approach, the agency was able to pinpoint errors in automation triggers and correct them with minimal downtime. This case study showcases how detailed performance analytics can drive tangible improvements in productivity.
Other Industry Examples
Other companies have also successfully integrated evaluation metrics. For instance, a startup automating lead generation through HubSpot and LinkedIn implemented regular reviews of its AI agent's performance. The key metrics included:
- Lead retrieval accuracy
- Conversion rate improvements
- User feedback scores
These companies report that consistent performance monitoring leads to better decision making and ultimately higher revenue. For more insights on building a robust evaluation model, consider reading through the article on transforming modern businesses and checking examples on the step-by-step guide. This shows that regardless of industry, a structured framework for evaluation and adjustments based on metrics is key.
Integrating Evaluation Metrics into Your Automation Workflow
Once you understand what to measure, it is critical to integrate these metrics within your automation strategy. Incorporating evaluation as a regular part of your workflow means you can catch potential issues before they become significant problems. This proactive approach is essential for keeping your systems aligned with business needs.
Adopting a Continuous Feedback Loop
A continuous feedback loop enables you to make improvements in real time. Begin by setting up regular review sessions to analyze metrics such as task execution speed, error rates, and user satisfaction. With the feedback loop in place, you can adjust parameters and enhance system performance continuously.
Implementing Evaluation in Daily Operations
Below are some implementation steps you should consider:
- Schedule weekly and monthly audits to review performance data.
- Create dashboards that centralize your key metrics.
- Incorporate both automated analytics and user surveys to gather performance insights.
- Set up alerts for anomalies so that you can address issues immediately.
This structure ensures that every stakeholder is aware of current performance levels and that continuous improvements are part of everyday operations. To see further examples of integrating workflows, check the Asana integration or explore details on the Google Sheets integration page.
Final Thoughts and Steps Forward
Effective ai agent evaluation is more than a technical requirement—it is the cornerstone of developing reliable, user-friendly, and efficient automation solutions. Throughout this discussion, you have learned how key metrics like response time, error rate, and user satisfaction can be harnessed to optimize performance. Establishing clear frameworks and incorporating data-driven continuous improvements are crucial steps to success.
Looking at real-world success stories, from digital agencies optimizing multi-tool tasks to startups refining lead generation, it is clear that incorporating robust evaluation methods leads to tangible benefits. By integrating these practices into your workflow, you elevate not only the efficiency of your AI agents but also the overall outcomes of your automated systems.
If you are ready to take your operations to the next level, consider experimenting with these evaluation methods and monitoring improvements over time. You can sign up for a platform that offers advanced multi-agent AI capabilities, ensuring that your workflows meet industry standards. For ready-to-use automations, visit the marketplace and explore prebuilt automation solutions that have been refined through rigorous evaluation practices.
Remember, regularly reviewing and updating your set parameters is key. As you adapt to evolving needs, combining these metrics with user insights will help you stay ahead of the curve. For more practical tips on advanced integrations and productivity tools, visit the main page at Assista or explore additional resources available on our blog. Embrace a culture of measurement and continuous improvement to ensure your automated solutions deliver real value.
Join over 1,400 professionals who reclaimed their time and sanity by letting AI handle the busywork.