AI Agent vs RAG: Comparing Core Technologies
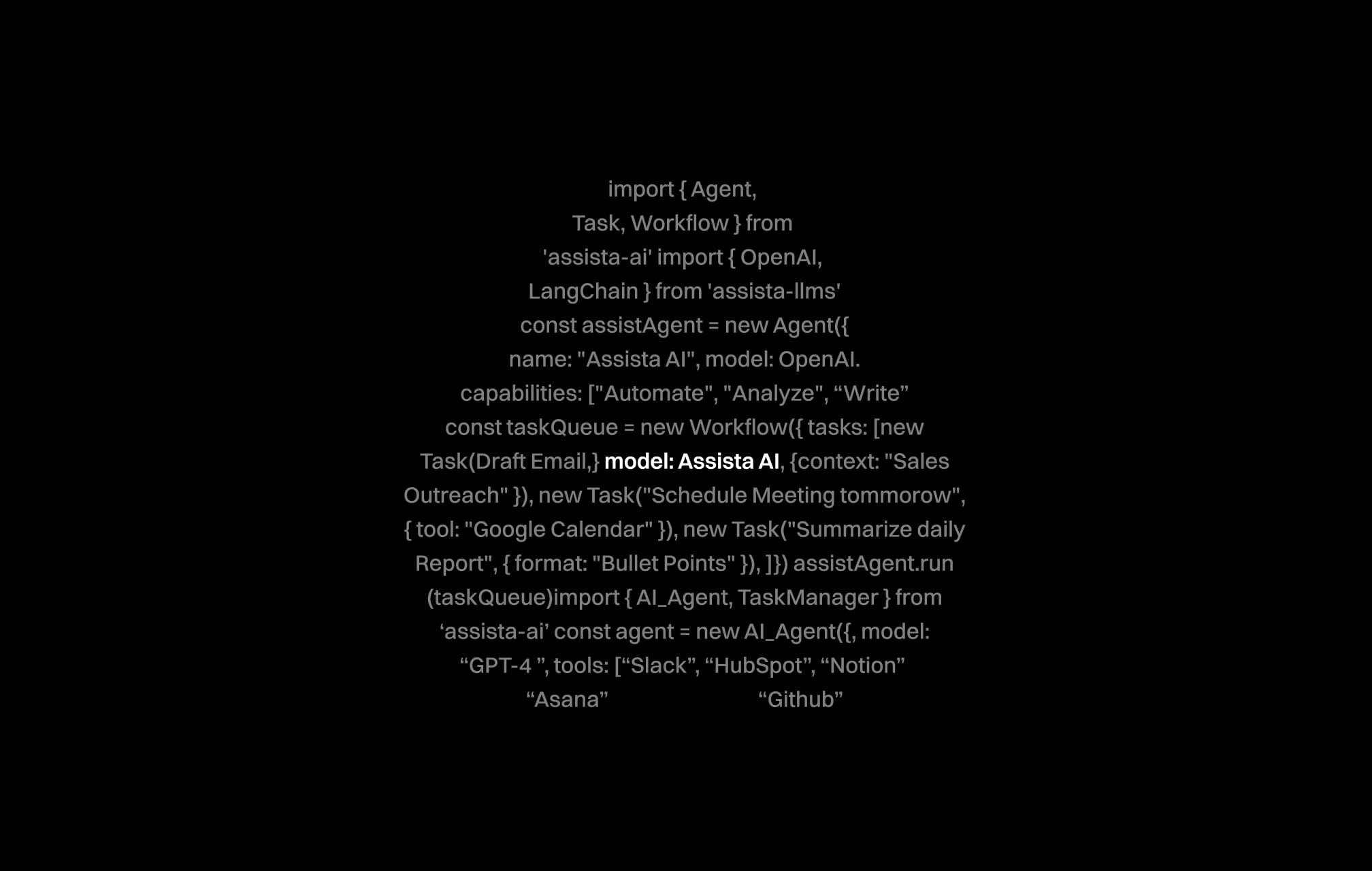
The Landscape of Intelligent Data Processing
Are you trying to decide between an AI Agent and a RAG system for handling your data processing tasks? You are not alone in your quest for a solution that can manage complex workflows while delivering accurate and timely insights. As more organizations turn to intelligent automation, understanding the distinct capabilities of these two approaches becomes crucial. In this article, we explore the mechanics of AI Agents and RAG systems, comparing their core technologies and real-world applications. You will learn about the benefits and limitations of each approach, how they integrate with popular tools like Gmail, Slack, and Google Sheets, and what the future might hold. By the end, you can make a more informed decision that aligns with your specific workflow needs.
This guide is ideal for professionals without extensive technical expertise who are looking to enhance productivity through smart automation solutions. Whether you’re a digital worker, a startup leader, or part of a large enterprise, understanding the differences between AI Agent vs RAG is essential. Let’s delve into the world of intelligent automation and examine the foundations that make these technologies unique, starting with the basics.
Understanding AI Agents
AI Agents are designed to simulate human task execution in digital workflows. They excel at orchestrating multiple, often complex, actions across various platforms in real time. For example, platforms like Assista work with numerous productivity tools such as Gmail, Slack, HubSpot, Notion, and Trello to transform natural language commands into coordinated multi-step operations. Data suggests that businesses leveraging integrated AI solutions have seen significant improvements in productivity and operational accuracy.
An AI Agent’s core value lies in its ability to:
- Fetch data from diverse sources
- Generate actionable insights
- Handle decision-making processes
- Execute tasks seamlessly on multiple applications
To support this functionality, many platforms integrate AI Agents with advanced orchestration tools and workflows. For instance, if you need to update customer records in Salesforce while simultaneously generating a report in Google Sheets, an AI Agent can coordinate this through a natural language chat interface.
For more detailed insights into the role of AI Agents, check out the article on AI agents explained from concept to execution on our website. Next, we will explore the nuances of RAG systems and how they differ from traditional AI Agents.
Decoding Retrieval Augmented Generation (RAG) Systems
While AI Agents focus on automating and executing tasks across various platforms, RAG systems emphasize enhancing data processing through augmented retrieval. RAG, or Retrieval Augmented Generation, combines the generative capabilities of modern language models with robust retrieval components. These systems fetch relevant documents or data points to provide more contextually accurate answers or insights.
The benefits of RAG systems include:
- Improved accuracy by grounding responses in real data
- Enhanced contextual understanding
- Reduced hallucination in generated content
- Flexibility to incorporate diverse data sets
In scenarios where precise data and context are critical, RAG systems can outperform traditional generative models. A notable example is their use in research and legal document analysis, where every detail counts and precision is key. Moreover, RAG systems have been successfully implemented in customer support and knowledge base management. This approach efficiently pairs the power of a language model with the accuracy of stored data.
You can learn more about integrated solutions by exploring our extensive integrations page, which showcases how Assista effectively connects various tools to supercharge productivity.
AI Agent vs RAG: A Comparative Analysis
Comparing AI Agents with RAG systems brings several considerations to the forefront. To help you navigate these technologies, we have broken down their core components and examined the advantages and limitations of each approach.
This comparison focuses on these key areas:
- Functionality and Capabilities
- Accuracy and Reliability
- Integration with Existing Systems
- Scalability and Flexibility
Functionality and Capabilities: AI Agents are exceptional for multi-step workflow execution. They not only automate repetitive tasks but also integrate with over tens of productivity tools. RAG, however, shines when the need is for contextual data retrieval. For example, if your work revolves around analyzing spreadsheets and generating actionable insights, the generative component in RAG systems helps provide the right data points for better decision-making.
Accuracy and Reliability: RAG systems typically offer increased accuracy due to their ability to reference real-time data during generation. In contrast, while AI Agents excel at coordination, they may depend on the quality and structure of the integrated data sources to perform optimally.
Integration with Existing Systems: One of the biggest challenges is aligning new technology with familiar workflows. AI Agents can easily integrate with various platforms such as Gmail, Google Sheets, and Slack. This makes them highly attractive for professionals without a technical background. RAG systems, on the other hand, require more sophisticated indexing and retrieval architectures to merge with existing databases effectively.
Scalability and Flexibility: When your workload grows, scalability becomes a top consideration. AI Agents are scalable in terms of task coordination and workflow management, particularly in platforms like Assista that accommodate thousands of actions across various tools. RAG systems scale by expanding the scope of their retrieval databases, ensuring that the data driving responses remains current and relevant. Each system has its strengths and weaknesses, so the choice depends on your particular needs, whether it's multi-step workflow execution or enhanced contextual data retrieval.
Both systems are continuously evolving. For an external perspective on the evolution of intelligent data solutions, take a look at Gartner, which provides valuable insights and trend analysis.
This breakdown should help you appreciate the trade-offs between the two technologies. Next, we explore how these differences translate into practical applications, and which use cases are ideal for each technology.
Practical Applications: Making the Right Choice for Your Workflow
Selecting the right technology is about understanding your own workflow needs. Whether you lean towards the control and orchestration provided by AI Agents or the targeted data retrieval offered by RAG systems, each has its ideal application scenarios.
Consider the following when deciding between the two approaches:
- If your work involves managing complex tasks across multiple tools like Notion, Asana, and LinkedIn, AI Agents can coordinate these seamlessly. They are ideal for automating processes like lead generation, customer support, and performance reporting.
- If your focus is on guaranteeing data accuracy and contextual relevance—such as in academic research, legal documentation, or detailed market analysis—RAG systems may provide the necessary precision.
A case study from a mid-sized digital agency illustrates this well. The agency utilized an AI Agent platform to automate task management and client communications across Trello and Slack. They achieved a 30% improvement in workflow efficiency without a steep learning curve. In another instance, a research firm adopted a RAG system to enhance accuracy in their data analysis workflow, resulting in more reliable reports and actionable business strategies.
For professionals ready to explore these advanced workflows, consider using our platform. You can sign up today and start leveraging AI Agents to automate your most time-consuming tasks. Additionally, extra tools and prebuilt automations are available in our marketplace.
Other internal resources that may help include our about page, our pricing details, and our comprehensive changelog to keep you updated on the latest improvements. These links provide valuable context on how evolving digital workflows can be transformed using targeted technology.
Future Trends and Strategic Considerations
The technological landscape is ever-changing. Both AI Agents and RAG systems are at the forefront of innovation, and future advancements promise even greater levels of efficiency and precision. Emerging trends include:
- Enhanced multi-agent collaboration, where different AI components work together to solve tasks more holistically
- Greater integration of natural language processing to reduce manual intervention
- Expansion into new industries such as healthcare, finance, and legal services for both workflow automation and data retrieval
The strategic choice between AI Agent vs RAG will depend on multiple factors including the complexity of your workflows, the volume and variety of your data, and your desired outcomes. By staying informed of industry trends through resources like our expert articles and external publications such as those from Harvard Business Review, you can anticipate valuable shifts in the landscape.
Moreover, decision-makers need to consider scalability. Over time, you might start with AI Agents to get tasks automated and later integrate RAG components when working with larger, more complex data sets. This evolutionary approach can be supported by flexible platforms that offer integrations with tools like GitHub and Google Calendar.
By understanding the trajectory of these technologies, you position your organization to benefit from continuous innovation. The competitive edge lies in not only choosing the right tool but also in knowing when to evolve your strategy. Such strategic planning ensures that your workflows remain adaptive and productive.
Wrapping Up Your Decision-Making Process
In summary, the debate between AI Agent vs RAG centers on two pillars: workflow orchestration and data retrieval accuracy. AI Agents excel in coordinating tasks across multiple applications and reducing manual burdens, making them perfect for everyday digital operations. In contrast, RAG systems offer enhanced precision by augmenting data generation with real-time retrieval, thus providing more context-rich outputs.
You now understand that the choice between these two technologies depends on your specific needs. If your goal is to automate and manage multi-step processes across platforms such as Gmail, Slack, and Trello, leveraging a platform like Assista will empower you to automate tasks without requiring technical expertise. On the other hand, if your focus is on ensuring the highest levels of accuracy and contextual data usage, adopting a RAG approach may be ideal.
This comprehensive comparison should help you navigate these options. Are you ready to boost your productivity and make smarter decisions about data processing? Consider the future of intelligent automation by evaluating your workflow needs, and remember that integrating intelligent tools can transform not just processes, but overall business operations. With smart automation technologies evolving continuously, now is the perfect time to explore and adopt a solution that bridges the gap between possibility and practicality.
If you have further questions or need personalized guidance, feel free to visit our contact page or check out our extensive educational resources, including detailed guides on effective integrations. Make a confident decision today by harnessing the remarkable potential of these technologies and propel your digital workflows forward.
Join over 1,400 professionals who reclaimed their time and sanity by letting AI handle the busywork.