AI Agent vs LLM: Evaluating Automation and Language Processing
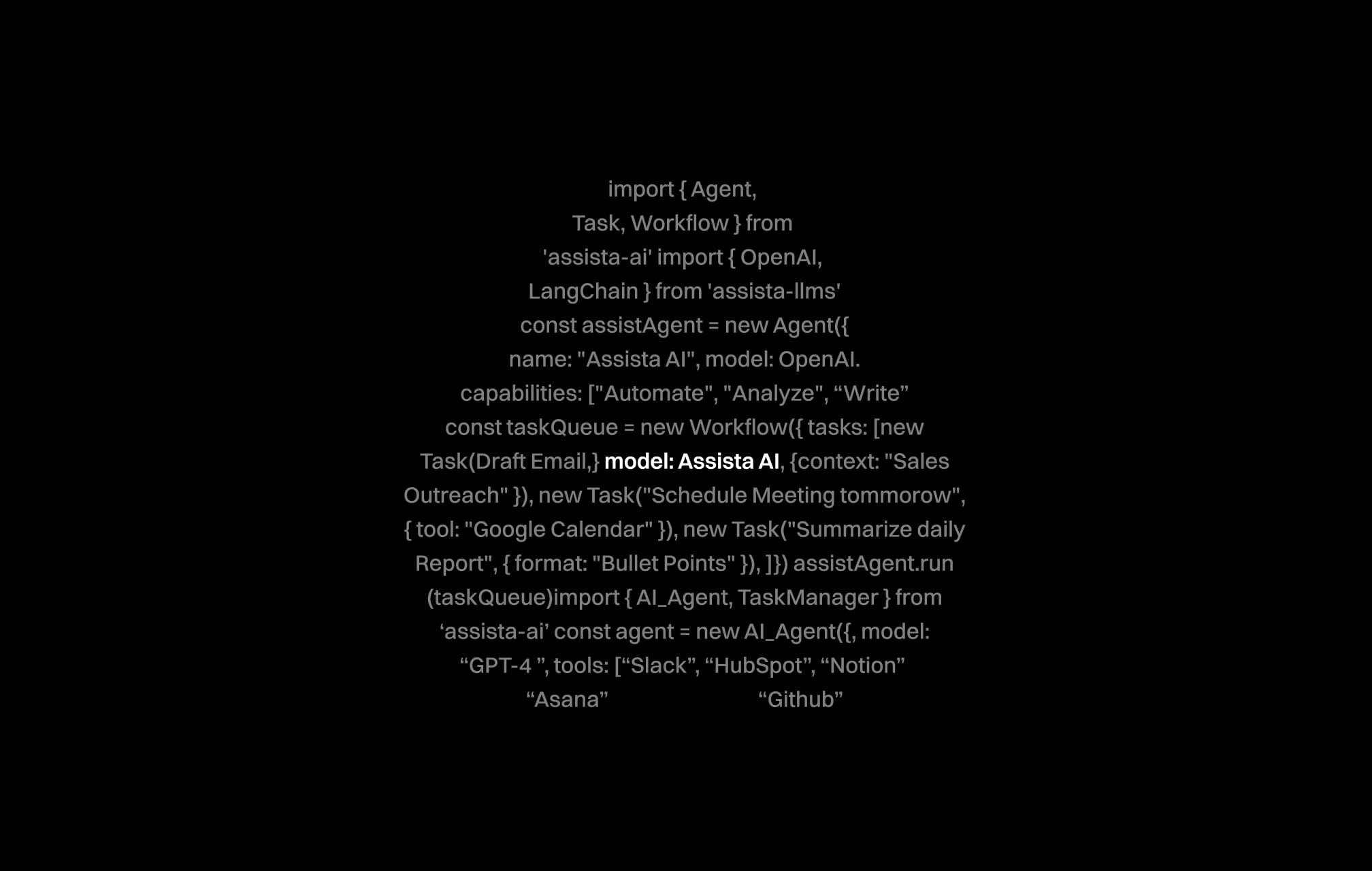
Understanding the Basics
If you have ever wondered whether to deploy an AI Agent or a Large Language Model (LLM) for your workflow, you are not alone. Many professionals face the challenge of selecting the right tool for automation and language processing. This article delves into the differences between an AI Agent and LLM and explains how each can contribute to efficient task management. By comparing their functionalities, you will gain clarity on which option aligns best with your business needs and goals.
In today's technology-driven work environment, the choice between these two powerful technologies matters. Whether you need to execute multi-step processes across platforms like Gmail, Slack, or Google Sheets, or simply wish to leverage sophisticated language processing, understanding these tools is critical. We will explore definitions, core differences, practical applications, and best practices for adopting each solution. This comprehensive guide will help you decide which strategy is most beneficial for enhancing your productivity.
Foundations: What Are AI Agents and LLMs?
Clarifying what an AI Agent and a Large Language Model (LLM) are is essential before dissecting their unique applications. This section highlights their key characteristics and roles in modern automation and language processing.
Defining AI Agent
An AI Agent is designed to execute tasks by integrating and orchestrating multiple applications. For instance, Assista connects with tools such as Gmail, Slack, HubSpot, Notion, and many others. This platform leverages multiple AI Agents working side-by-side to execute actions like sending emails, retrieving data, updating tasks, or analyzing spreadsheets. AI Agents focus on automation, coordinating multi-step workflows to convert natural language prompts into real actions.
Statistics show that companies using automated agents can reduce manual processing time by up to 40%. Here are some key benefits of adopting AI Agents:
- Improved cross-platform coordination
- Reduction in repetitive manual tasks
- Enhanced workflow efficiency
Learn more about integrating multiple tools by visiting the integrations page.
Defining Large Language Models (LLMs)
Large Language Models are trained on massive amounts of text data, enabling them to understand, generate, and manipulate language. Unlike AI Agents that focus on task automation, LLMs process natural language queries and provide contextually appropriate responses. They are used in writing assistance, customer support, and content creation.
This technology leverages probability models to predict the next word or sentence, making LLMs excellent conversational partners. According to recent studies published on Nature, LLMs have revolutionized language-based tasks by processing and generating text in real time. Some defining features include:
- Contextual understanding of language
- Generation of human-like responses
- Support for a wide range of language applications
For further reading on advanced language models, you may check research articles on ScienceDirect.
Core Differences in Functionality and Automation
This section explains the primary distinctions between AI Agents and LLMs. While they share similarities in processing natural language, their roles diverge significantly when it comes to automation and task execution.
Targeted Capabilities
AI Agents are highly focused on executing pre-defined workflows. Their integration with productivity tools enables tasks such as sending out emails, updating customer records in HubSpot, or generating performance reports. In contrast, LLMs excel at interpreting and generating text, making them better suited for scenarios that require nuanced language understanding or creative input.
When evaluating the ai agent vs llm debate, key differences include:
- Functionality: AI Agents trigger specific actions across multiple platforms, while LLMs provide text-based support and conversation.
- Automation: AI Agents manage multi-step processes seamlessly by interacting with systems like Asana, Jira, or Salesforce; LLMs focus on understanding and language generation.
- Operational scope: AI Agents are task-oriented and efficient at repetitive functions, whereas LLMs are versatile in understanding context and generating insightful responses.
This differentiation is paramount if your primary objective is cross-platform automation versus language processing. Understanding these capabilities helps in making an informed decision.
Integration with Existing Tools
AI Agents typically incorporate integrations with various enterprises tools. For example, a successful use case involved automating customer support ticket routing between Zendesk and Linear. Companies implementing such solutions have observed reduced response times and more organized workflows.
On the other hand, LLMs are more frequently adopted for creative and communication-centered tasks, such as drafting marketing content or generating social media posts.
For a deeper dive into automation scenarios and real-world examples, visit the changelog page where updates and case studies are shared.
Real-World Applications and Use Cases
One of the core advantages of understanding the ai agent vs llm discussion is recognizing their distinct real-world applications. This section examines practical uses and case studies that demonstrate how each technology drives business outcomes.
AI Agent Use Cases
Consider a scenario where your team uses multiple tools like Gmail, LinkedIn, and HubSpot. An AI Agent can automatically send emails, retrieve leads from HubSpot, and update records simultaneously. This active orchestration not only saves time but also reduces errors caused by manual intervention.
Some common examples of AI Agent applications include:
- Customer support ticket management
- Automated social media postings
- Lead generation from LinkedIn and HubSpot
A notable case study involved a mid-sized enterprise that deployed an AI Agent solution to manage cross-platform workflows. Their efficiency increased by 35% as routine tasks were executed automatically. Additionally, the company integrated systems such as Trello and Slack for better communication and task tracking. The success of this strategy is discussed further in a related article on how AI Agents are transforming modern businesses.
LLM Use Cases and Creative Applications
LLMs are predominantly used in situations where dynamic language capabilities are required. Marketers often use LLMs to generate compelling ad copy or product descriptions. Customer support teams benefit from the language understanding skills of LLMs by providing quick, accurate responses to client inquiries.
Examples of LLM usage include:
- Content generation for blogs, social media, and websites
- Answering customer queries in chat interfaces
- Assisting in drafting and editing reports or proposals
Both use cases highlight the impact of choosing the right tool. When the focus is on generating nuanced textual content, LLMs shine; however, for executing multi-step procedural tasks, AI Agents are the better choice.
Best Practices for Implementation
Implementing either an AI Agent or LLM solution requires thoughtful planning. In this section, we outline best practices and strategies to ensure that you select and deploy the tool that aligns with your objectives.
Assess Your Business Needs
The selection process begins with a detailed analysis of your business requirements. Consider the following factors when choosing between an AI Agent and LLM:
- Are you looking to automate repetitive tasks across multiple platforms?
- Do you require sophisticated language processing and creative content generation?
- What are your integration needs with existing tools like Google Sheets, HubSpot, or Salesforce?
Evaluating these criteria will help you decide which tool better serves your objectives.
Design Robust Workflows
Once you have identified your needs, design your workflows accordingly. For AI Agents, this might involve mapping out each step of a multi-app process. For LLMs, defining clear prompts and expected outcomes is key. Remember that well-thought-out workflows reduce errors and improve efficiency.
Practical tips for workflow design include:
- Documenting each step for clarity
- Testing workflows with a smaller team before organization-wide implementation
- Monitoring performance regularly and refining processes
For a detailed walkthrough on creating effective automation workflows, check out the step-by-step guide to build an AI Agent.
Security and Compliance
It is essential to ensure that your automation processes adhere to security standards. When integrating solutions with platforms such as Microsoft Teams or LinkedIn, always review security protocols to prevent data breaches. Using a trusted platform like Assista, you benefit from robust security measures already in place. For additional reassurance, you may also review details on the about page.
Future Outlook and Strategic Considerations
Looking ahead, both AI Agents and LLMs will continue to evolve, opening new pathways for automation and communication. Companies will increasingly blend the strengths of both approaches to create hybrid systems that deliver enhanced outcomes.
Embracing Hybrid Solutions
Rather than viewing AI Agents and LLMs as mutually exclusive technologies, many innovators are exploring hybrid models. Such solutions combine the real-time task execution of AI Agents with the language processing prowess of LLMs. This approach helps organizations handle complex workflows while ensuring rich, context-sensitive communication.
Important strategic considerations include:
- Evaluating cost-effectiveness and potential ROI
- Considering scalability as your organization grows
- Adapting workflows to incorporate emerging trends and capabilities
Decision-makers must analyze both short-term needs and long-term growth projections. Articles such as innovative AI Agent business ideas to boost growth offer additional insights into planning and strategy.
Monitoring Industry Trends
The technology landscape is dynamic, and staying informed is key. Monitor industry reports, case studies, and expert opinions to remain competitive. Adopting an iterative approach to technology implementation will allow your business to pivot effectively as new innovations emerge.
For example, several businesses have successfully transitioned to hybrid systems and reported increased productivity and customer satisfaction. To explore more on AI technologies and their impact on business operations, visit our blog.
As you weigh the benefits between AI Agent and LLM, consider both the immediate and future value each solution provides. Each strategy has distinct advantages that can be tailored to your operational needs.
Ready to revolutionize your workflow? If your primary focus is on automating complex, multi-step tasks across your favorite productivity tools, why not sign up with Assista today? In addition, explore pre-built automations on the marketplace to see how others are leveraging these technologies for their own success.
In summary, the competition between AI Agent and LLM is not about which technology is superior, but rather which one aligns best with your organizational needs. By understanding the core differences in functionality, integration capabilities, and application best practices, you can make an informed decision that will enhance your work processes. Embrace the future by choosing the tool that drives your productivity and adapts to evolving business challenges. Your journey to smarter automation and efficient language processing begins with an informed choice. Whether you opt for the structured task execution of AI Agents or the dynamic language prowess of LLMs, each path offers significant opportunities to boost operational efficiency and drive innovation. It is time to harness these technologies and redefine the way your team works.
Join over 1,400 professionals who reclaimed their time and sanity by letting AI handle the busywork.