AI Agent vs Agentic AI: Understanding Automation Approaches
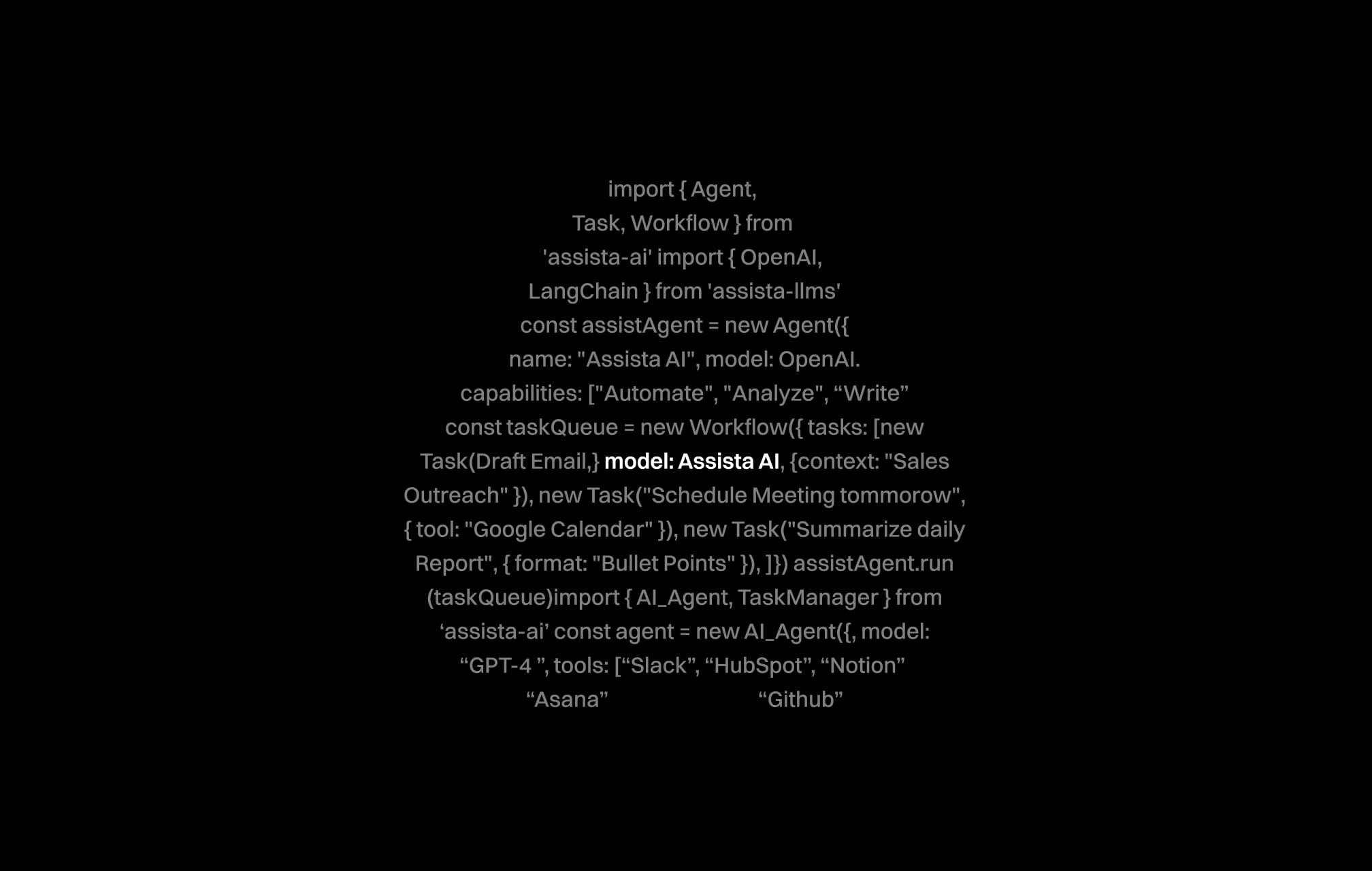
Are you trying to figure out which approach best fits your business needs?
Many digital workers wonder how conventional AI agents differ from the evolving concept of agentic AI when tackling complex tasks. If you’ve ever asked yourself about the differences between an ai agent vs agentic ai, you’re not alone. Understanding these approaches is crucial in a world where automation drives efficiency. In today’s landscape, businesses depend on various systems such as Gmail, Slack, HubSpot, Trello, and Google Sheets to manage hundreds of daily tasks. Yet, bridging these systems via automation can be challenging without a clear comprehension of each method.
This article takes a deep dive into both conventional AI agents and agentic AI. We’ll break down their definitions, examine how each framework powers task automation, and compare the distinct benefits and challenges involved. You will also learn about successful real-world applications and explore how Assista transforms digital workflows without needing technical expertise. Whether you manage a startup or a mid-sized company, knowing which approach fits your operational style could be the game-changer you need.
Defining AI Agent and Agentic AI
This section clarifies what distinguishes a conventional AI agent from agentic AI. Conventional AI agents are built to process and execute straightforward commands. They follow pre-programmed instructions to complete tasks across various platforms like Gmail or Salesforce. In contrast, agentic AI works with a degree of autonomy, allowing it to make decisions, fetch data, and even adjust its behavior based on the situation at hand.
Key Characteristics
When comparing these two, consider the following aspects:
- Decision-making: While AI agents work on a fixed set of rules, agentic AI is designed to assess context and choose optimal actions.
- Interactivity: Agentic AI can often interact with multiple systems at once, coordinating complex multi-step workflows.
- Adaptability: Agentic AI evolves with the task requirements and can incorporate feedback to modify its actions in real time.
For further insights into how these platforms can be integrated into your business model, you might explore details on the about page or learn how they are applied in different workflows on the integrations page. With this foundational understanding, you can appreciate how both methods bring value to modern operations, setting the stage for a deeper exploration of their implementation.
How Each Approach Tackles Task Automation
This part dives into the mechanics of how the ai agent vs agentic ai models tackle task automation. Each approach offers a unique method to problem solving and data management. Conventional AI agents excel in predictable, repetitive tasks. Their rule-based execution is reliable when tasks are well-defined. Conversely, agentic AI is better suited for scenarios where decision-making requires flexibility and continuous learning.
Step-by-Step Task Execution
Consider a scenario where your team needs to update client data across multiple apps. Here’s how the two models differ:
- Data Retrieval: A conventional AI agent queries a specific database and retrieves client records. Agentic AI not only fetches these records but also assesses if the data meets current business requirements.
- Data Analysis: The traditional agent performs pre-set analysis, while agentic AI evaluates multiple parameters to provide context-sensitive insights.
- Action Execution: The conventional model executes a fixed series of updates across platforms. Agentic AI can adjust the sequence or logic based on feedback it receives during the process.
Notably, external research such as studies published by McKinsey highlight how adaptive systems lead to improved operational performance. With this in mind, understanding whether your tasks fit a routine model or require nuanced decision-making can help you choose the right approach.
Automation Across Platforms
Multi-tool integrations are central to modern workflows. For instance, when managing campaigns that span LinkedIn, HubSpot, and Google Sheets, conventional agents execute defined sequences. On the other hand, agentic AI dynamically determines next steps based on real-time data, ensuring the entire process is more responsive. This capacity to interact fluidly with various tools is why platforms like integrations and even detailed use cases on the blog resonate with many businesses.
Transitioning to the next section, let’s explore the tangible benefits and considerations you should keep in mind when choosing between these technological approaches.
Comparing Benefits and Challenges
Understanding both the advantages and the limitations of conventional AI agents and agentic AI is key to optimizing your workflow. The ai agent vs agentic ai debate is multifaceted, with each method offering distinct strengths suited to different operational needs.
The Upsides
Here is a comparative look at the benefits each model delivers:
- Conventional AI Agents:
- Reliability through predetermined instructions
- Consistency in executing repetitive tasks
- Simplicity in design and implementation
- Agentic AI:
- Enhanced decision-making through context analysis
- Flexibility to adapt workflows in real time
- A more holistic approach to multi-step processes
Potential Drawbacks
While each system has benefits, there are challenges to consider as well:
- Conventional AI Agents:
- Limited adaptability when encountering unexpected scenarios
- Dependency on clearly defined parameters
- Agentic AI:
- Complexity in implementation and higher resource requirements
- The need for more robust data inputs to achieve accurate decision-making
Internal pages such as the changelog provide insights into continuous improvements, and the pricing page lets you explore how these solutions are scaled cost-effectively for various business sizes. The key takeaway here is that the value of each system lies in its suitability for the task at hand. Transitioning now, we will examine how these theoretical benefits translate into real-world applications and case studies.
Real-World Applications and Case Studies
Understanding theory is important, but seeing practical applications helps bring the differences between these approaches into focus. Organizations around the globe rely on both conventional AI agent models and agentic AI to automate cross-platform workflows and manage data-intensive tasks.
Case Study: Customer Lead Management
Imagine a mid-sized business handling lead generation and follow-ups via LinkedIn and HubSpot. A conventional AI agent is set up to retrieve leads from HubSpot at a scheduled interval, write emails through Gmail, and update status on Trello. This process is consistent, reliable, and minimal in required adjustments. However, unexpected changes in lead quality or scheduling conflicts may necessitate manual interventions.
Case Study: Adaptive Marketing Campaigns
Consider another scenario where a digital marketing team leverages agentic AI for a dynamic campaign. The system automatically pulls the latest campaign data from Google Sheets, analyzes performance trends, adjusts messaging based on customer behavior, and even recommends retargeting strategies. Here, the agentic model significantly reduces the need for constant monitoring and manual adjustments. Research by McKinsey suggests that companies with adaptive systems can boost operational efficiency by as much as 30%.
Key Takeaways from Implementations
Businesses leveraging these technologies often highlight the following advantages:
- Reduction in manual workloads
- Improved accuracy in task execution
- Enhanced agility in responding to market trends
On the flip side, organizations have reported that agentic AI may require higher initial investments and careful calibration to ensure that autonomous decision-making aligns with company standards. To further explore how different industries are addressing these challenges, visit the blog.
Integrating AI Agents into Your Workflow with Assista
This section explains how you can integrate automation into your everyday operations using Assista. Designed for non-technical digital workers, Assista brings together AI agents that work in real time to transform your natural language prompts into coordinated, multi-step workflows. With Assista, you do not need to be a tech expert to command complex actions across multiple tools.
Getting Started is Simple
Assista connects with many popular tools including Gmail, Slack, HubSpot, Notion, Asana, Trello, Google Sheets, Salesforce, Jira, and LinkedIn, among others. With the power of multiple AI agents collaborating, you can:
- Automatically send emails
- Retrieve valuable HubSpot leads
- Update and analyze tasks and reports
- Create and post marketing content
To start reaping these benefits, you can sign up and begin automating your workflows immediately. Moreover, the Assista Marketplace provides an ever-growing selection of prebuilt automations. Check out the marketplace for ready-to-deploy solutions that many businesses have already adopted to optimize their work processes.
Ensuring a Smooth Transition
Before adopting either conventional AI agents or agentic AI, assess your team’s specific requirements. Consider the following practical steps:
- Identify repetitive tasks that consume a lot of time.
- Determine whether these tasks are predictable or require adaptive responses.
- Evaluate current integrations and platforms used by your team.
- Test with small-scale automations available on the marketplace.
- Scale up as your comfort and results grow.
This approach aligns with best practices discussed on the blog and offers a real-world blueprint for transitioning to automation solutions. By meticulously assessing your needs, you can select an automation strategy that not only saves time but also enhances your team’s overall productivity.
Wrapping Up Your Automation Journey
The exploration of ai agent vs agentic ai reveals that while conventional AI agents are designed for reliability and consistency, agentic AI holds significant promise for adapting to dynamic environments through its decision-making and multi-agent collaboration. Both approaches have unique advantages that, when thoughtfully applied, can transform your digital workflows.
As you consider the best automation strategy for your business, keep these points in mind:
- Understand the task complexity: Repetitive, predictable tasks are best suited for conventional AI agents.
- Embrace adaptability: If your operations require real-time decisions based on contextual data, agentic AI may be the way forward.
- Evaluate the cost-benefit: Although agentic AI may require a larger upfront investment, the efficiency gains over time are often substantial.
To sum up, by analyzing the differences in how these systems operate, you gain a clearer picture of which one aligns with your workflow challenges and business goals. Whether you opt for the reliability of conventional AI agents or the sophistication of adaptive, agentic AI, the ultimate aim is to empower your team to focus on what matters most.
Now is the time to take charge of your digital operations. Explore the power of automation with Assista and discover how advanced multi-agent AI can elevate your everyday work. Begin by exploring our integrations, checking out our affordability on the pricing page, or reading more on our blog to stay ahead in this automation revolution. Your next step toward enhanced productivity awaits.
Join over 1,400 professionals who reclaimed their time and sanity by letting AI handle the busywork.