AI Agent Memory: Enhancing System Performance with Data Recall
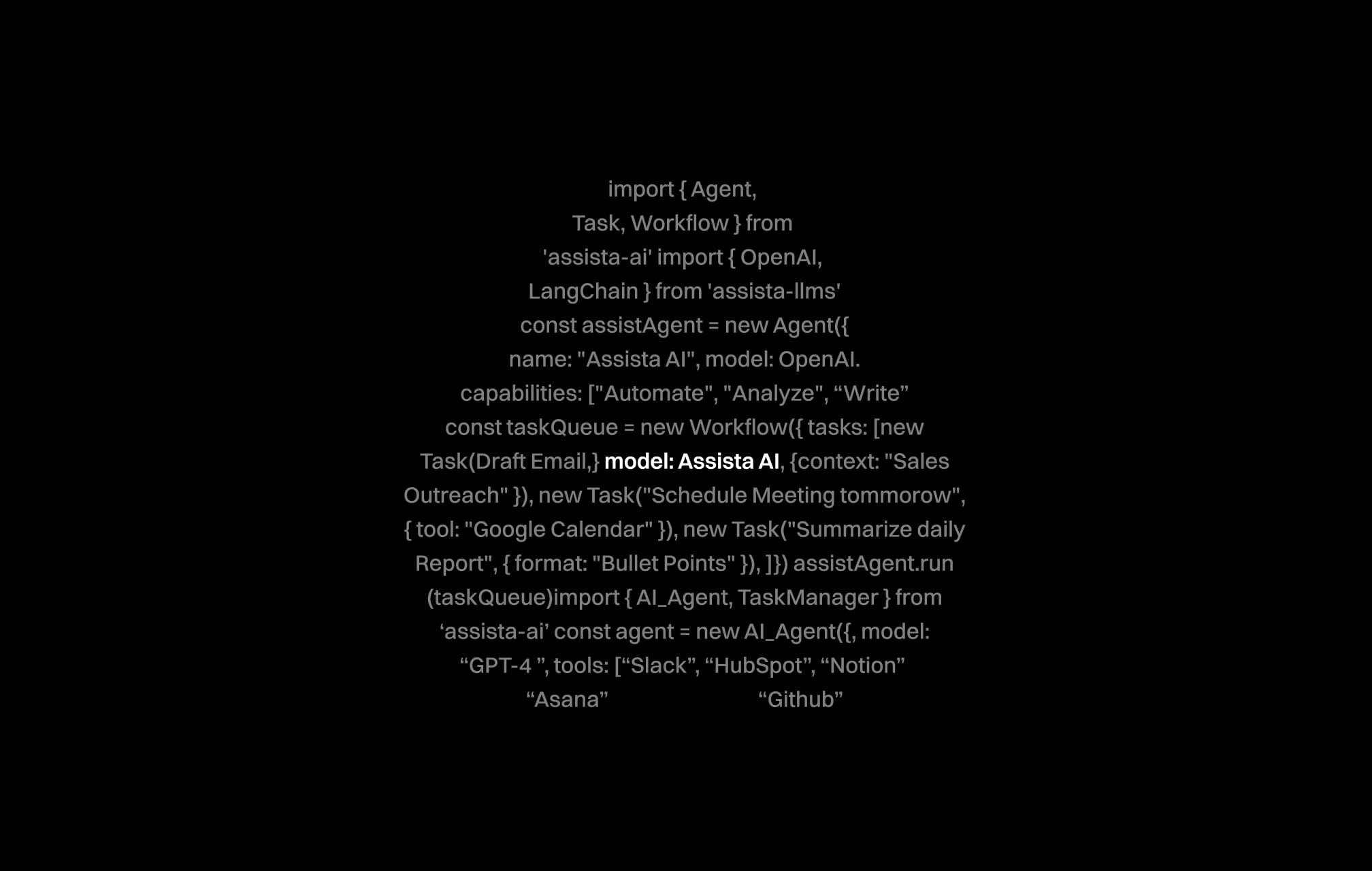
Unlocking the Potential of AI Agent Memory
In today's fast-paced digital environment, you may have wondered how systems can be optimized to remember past interactions and deliver better results. AI agent memory plays a pivotal role by retaining historical data and using it for improved decision-making. This article offers valuable insights into how AI agent memory elevates system performance through data recall and intelligent analysis. We discuss what AI agent memory is, how it boosts efficiency, and why integrating it into your workflow can be a game-changer. Whether you are a business leader searching for enhanced productivity or a digital worker looking for smarter automation, our deep dive covers technical details, real-world examples, and future trends that matter to everyone. As you read on, you will learn about the mechanisms behind data recall, best practices for deployment, and real-life use cases that demonstrate substantial improvements in system operations.
By exploring topics such as data storage mechanisms and integration across platforms like Gmail, Slack, and HubSpot, you'll see how critical retaining past interactions is for robust AI performance. We also highlight the strategic considerations needed to harness this technology effectively in everyday business processes.
Understanding AI Agent Memory
What Exactly Is AI Agent Memory?
AI agent memory refers to a system's ability to store and recall past interactions. When AI can bring historical context into discussions and ongoing tasks, it can tailor decisions and responses that are more personalized and timely. For example, digital workers often rely on historical data to prioritize tasks and accelerate decision-making. This is not just about storing data; it's about drawing actionable insights from it.
Consider a scenario where a marketing manager uses historical lead data to adjust campaign strategies. The ability to recall previous outcomes directly influences the efficiency of current operations. By incorporating memory into AI functionalities, businesses tap into an underlying wealth of information, transforming random data points into meaningful intelligence.
Why Memory Matters in System Operations
Memory in AI systems helps to improve performance by reducing the need for repetitive data gathering and analysis. When decisions are based on proven historical data, the margin of error decreases, and operational consistency increases. Additionally, retaining context is crucial for multi-step workflows, where each action depends on the previous one for continuity and accuracy.
This technology can be compared to a human's ability to learn from experience; the more interactions a system processes, the sharper its responses become. In fact, research from McKinsey & Company shows that organizations using data-driven strategies can see performance improvements of up to 20%.
Understanding AI agent memory is essential because it underpins many of the strategic functionalities found in modern productivity platforms. For more insights on this topic, check out our detailed guide at our integrations page which explores how various tools work together to create robust workflows.
Real-World Impact on System Efficiency
Enhancing Efficiency Through Data Recall
AI agent memory is not a theoretical concept; it delivers tangible benefits in real-world applications. By retaining information from prior interactions, digital workers can optimize processes in virtually every industry. For example, consider an operations manager who uses historical task data stored in AI agent memory to prioritize customer support tickets. The system automatically recalls context, improves response timing, and adjusts workflows without manual intervention.
This enhanced performance has multiple benefits:
- Increased response speed, as the system no longer reprocesses previous data.
- Improved decision accuracy with context-aware intelligence.
- Higher productivity by reducing repetitive tasks and boosting automation.
You can also imagine a sales team that leverages past interactions with leads. By having key details at their fingertips, they are better prepared for follow-ups and can personalize pitches more effectively. This leads to increased engagement and revenue growth.
In addition, the ability to link historical data with current actions helps organizations avoid pitfalls like data duplication and miscommunication. To further explore how these efficiencies are achieved across various platforms, visit our about page for a broader look at our technology philosophy.
Technical Aspects of AI Agent Memory
Data Storage and Retrieval Mechanisms
At the technical level, AI agent memory combines multiple data storage and retrieval techniques. Systems often use a blend of structured databases, caching methods, and real-time data processing engines to store and recall information effectively. These mechanisms ensure that historical data is not only stored securely but is also available instantly when needed.
Data recall comes from algorithms that index every interaction, ensuring that even complex multi-step processes are recorded accurately. The end result is a system that continually learns from every action, adapting its responses based on past successes and failures.
Key factors in these mechanisms include:
- Speed: Rapid data retrieval ensures responses are timely.
- Accuracy: High precision in recalling information minimizes errors.
- Scalability: Systems are designed to handle increasing amounts of data without degradation of performance.
For those curious about implementing these strategies within your workflows, our changelog page offers updates and technical insights about our evolving systems.
Integrating with Multiple Platforms
One of the greatest triumphs of AI agent memory is its seamless operation across various business applications. Modern productivity platforms like Gmail, Slack, HubSpot, Notion, Asana, Trello, Google Sheets, Salesforce, Jira, LinkedIn, and Webflow can all benefit from a memory system that delivers personalized outputs based on historical data.
For example, if you need to send a follow-up email or check past project updates, the system automatically references stored interactions. This integration saves time and reduces errors, enabling you to concentrate on strategic tasks instead of repetitive data entry.
Consider this quick checklist for integrating AI agent memory effectively:
- Identify key interactions to be stored, such as emails, task updates, and CRM entries.
- Configure data retrieval settings to ensure rapid access during multi-step workflows.
- Ensure all integrated applications support real-time data exchange.
- Regularly review and update memory protocols to align with business priorities.
For a full list of supported tools and further details on integration, you can visit our integrations page.
Best Practices and Key Use Cases
Strategies for Effective Deployment
Implementing AI agent memory within your system requires a thoughtful strategy that prioritizes both efficiency and security. The first step is understanding which data is critical for recall and ensuring that it is stored in a secure and scalable manner. Here are some best practices to follow:
- Define clear objectives for data retention – know what you need to recall and why.
- Test your system for speed and accuracy to verify that memory recall does not slow down operations.
- Regularly update memory architecture based on performance reviews and user feedback.
- Integrate data privacy protocols so that sensitive information is protected at all times.
An example of effective deployment is seen in a mid-sized marketing agency. After integrating AI agent memory, they observed a 30% reduction in operational delays and a noticeable improvement in client communications. Campaign data was analyzed, and subsequent actions were informed by past performance, leading to sharper and more targeted marketing strategies.
Illustrative Use Cases and Case Studies
Across industries, businesses are experiencing the benefits of AI agent memory firsthand. Here are two notable examples:
- Customer Support Optimization: A global e-commerce company implemented AI agent memory in their support system. By automatically recalling order histories and previous customer interactions, support agents were empowered to resolve issues faster and provide personalized solutions. The result was an impressive 25% boost in customer satisfaction ratings.
- Sales Process Enhancement: A B2B sales team integrated AI agent memory with their CRM tool, HubSpot. With instant access to historical client data, the team was able to follow up on leads with a tailored approach. This customization led to a 15% increase in conversion rates, demonstrating the value of memory-driven engagement.
Such cases highlight the real-world potential of AI agent memory to transform operational workflows. For more examples and detailed strategies on deploying these solutions, you might find insights on our ai agent automation best practices page and our how ai agents are transforming modern businesses article.
Future Trends and Strategic Considerations
Innovations Driving the Future of AI Agent Memory
Looking ahead, the evolution of AI agent memory is set to redefine the landscape of business operations. As machine learning models become more sophisticated, AI memory systems will not only recall data but will also predict future needs. This predictive capability could influence areas such as dynamic resource allocation and proactive workflow adjustments.
Future developments might include:
- Real-time sentiment analysis to adjust responses on the fly.
- Advanced anomaly detection to flag unusual patterns before they escalate.
- Cross-platform smart integrations that learn user habits and automate routine tasks without manual input.
In addition, businesses are likely to witness significant improvements in security and compliance around data recall systems. With more robust encryption and automated auditing, the next generation of AI agent memory will ensure that sensitive data remains confidential while driving efficiency.
To stay updated on new trends and technical advancements, you might review research reports on emerging technologies from Forbes and insights on Harvard Business Review. These resources provide valuable overviews of the strategic directions that AI and data retrieval systems are likely to take over the next decade.
Looking at the strategic angle, it is essential to plan for continuous learning and adjustment. Some trends to watch for include:
- Enhanced cross-correlation of historical data across even more diverse digital tools.
- More intuitive user interfaces that offer real-time feedback based on recalled data.
- Greater emphasis on predictive analytics in customer relationship management.
With these advancements, you are better positioned to transform not just single processes, but entire workflows. This holistic approach to remembering and leveraging past interactions ensures your systems empower your team continuously.
Moving forward, exploring these trends not only sets you up for future success but also offers immediate benefits by refining your current operations. If you want to experience these advantages firsthand, consider sign up for our platform and explore the wealth of integrated tools available.
Also, take a moment to browse our marketplace to see a range of prebuilt automations designed to boost operational efficiency and make everyday tasks more manageable.
In summary, the future of digital workflows is bright, underpinned by the evolving capabilities of AI agent memory. This technology is poised to continue delivering deeper insights, higher performance, and smarter decision-making across industries.
As you reflect on the key insights discussed throughout the article, you now have a comprehensive understanding of how leveraging past interactions through AI agent memory can enhance system performance. It is clear that storing and recalling historical data not only optimizes current operations but also paves the way for predictive, adaptive systems that improve over time. By following best practices and staying abreast of emerging trends, you can transform your workflow into a dynamic, intelligent ecosystem that consistently outperforms expectations.
If you are ready to harness the full potential of this technology, take action now. Explore the resources available, experiment with integrations across your favorite tools like Gmail, Slack, and HubSpot, and see first-hand how data recall can be a transformative asset for your business. Embrace the future of operational intelligence and watch your system performance soar while enjoying the tangible benefits of smarter, more efficient decision-making.
This article has shed light on the power of AI agent memory and its multifaceted impact on efficiency, integration, and predictive analytics. Apply these insights to optimize your workflow, and let historical data drive your strategic decisions for enhanced productivity and sustained growth. Your journey to a more intelligent, responsive system begins now.
Join over 1,400 professionals who reclaimed their time and sanity by letting AI handle the busywork.